Deciphering the Supply Chain Chessboard: The Science of Decision-Making in Risk Management
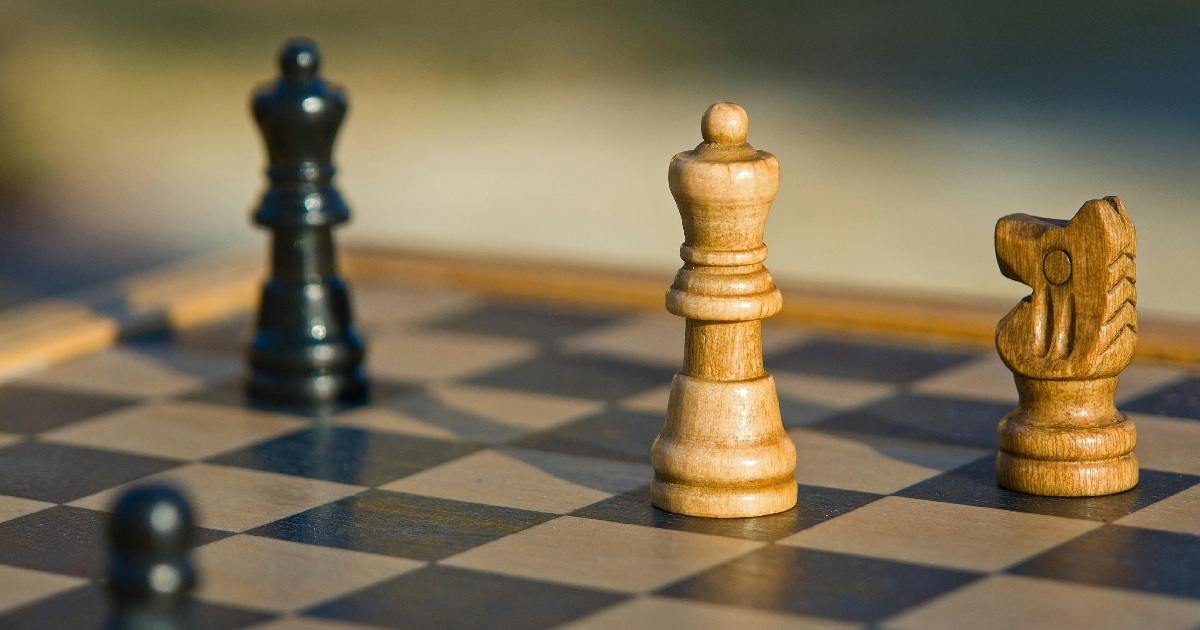
Dustin S. Sachs, DCS
Colorado Technical University, dustin.sachs@alumni.ctuonline.edu
https://doi.org/10.61643/c30815
Abstract
Humans make around 35,000 daily decisions, ranging from simple, routine choices to more complex, high-stakes ones (Pignatiello et al., 2020). In cybersecurity situations, decision-making accuracy is paramount, and emotions must be minimized to ensure objective choices are made (Korteling et al., 2023). Poor cybersecurity decision-making can have significant consequences, such as failure to protect sensitive data, prevent cyberattacks, or quickly respond to security incidents (Fischhoff & Broomell, 2020). The following qualitative study explored how these decision-making capabilities manifest in the cyber risk rating of third-party vendors, particularly under the influence of cognitive biases. The findings suggest that these cognitive biases can significantly impact the effectiveness of cybersecurity risk management. The study emphasizes the importance of addressing these biases to improve decision-making processes, suggesting that more objective and consistent evaluation methods could enhance the cybersecurity field's effectiveness and resilience.
Keywords: Cognitive biases, cybersecurity, third-party risk, risk assessment, decision-making
Recommended Citation
Sachs, D. S. (2024). Deciphering the supply chain chessboard: The science of decision-making in risk management. The Pinnacle: A Journal by Scholar-Practitioners, 2(2). https://doi.org/10.61643/c30815
Deciphering the Supply Chain Chessboard: The Science of Decision-Making in Risk Management
The SolarWinds cyber-attack of 2020 served as a stark reminder to organizations worldwide of the essential nature of their cyber supply chain (Perumannil & Haneef, 2021). The incident was a wake-up call for many organizations, as they discovered how vulnerable they were to cyber criminals who exploited their supply chains. SolarWinds, a leading provider of network monitoring software to thousands of organizations across various sectors, was the victim of a massive cyber-attack that compromised its software source code. The attackers then distributed the modified code automatically to SolarWinds customers, compromising an estimated 18,000 organizations. In the aftermath of the attack, nine US governmental agencies confirmed that data exfiltration from their networks had occurred (Perumannil & Haneef, 2021).
The SolarWinds incident highlighted the critical role that third-party vendors play in the cyber supply chain and the risks associated with their use. Organizations often rely heavily on third-party vendors to provide software and services for daily operations. However, these vendors often have access to sensitive data and networks, making them an attractive target for cybercriminals. The SolarWinds attack was a stark reminder that organizations must be aware of the risks associated with their supply chains and take steps to mitigate them.
Cognitive biases are pervasive in human decision-making and can significantly impact cybersecurity strategies and responses (Melnyk et al., 2022). In the context of supply chain-related threats, cognitive biases can be detrimental. Organizations may anchor their trust in a long-standing supplier, assuming their products or services are secure (Korteling et al., 2023). Anchoring bias could lead to a failure to thoroughly vet the security practices of that supplier, even when evidence suggests vulnerabilities or risks. Moreover, confirmation bias might make organizations reluctant to acknowledge potential security flaws in their supply chain (Korteling et al., 2023). Bias may lead to delayed action and increased exposure to threats like supply chain attacks, where malicious actors compromise a trusted supplier to infiltrate a target organization.
Study Problem
The problem addressed by this study is that cognitive bias in human decision-making leads to inconsistent and unreliable cybersecurity risk assessment results regarding potential third-party vendors, which results in poor decision-making (Carmichael, 2020; Cheung et al., 2021; Kahneman et al., 2021; McAlaney & Benson, 2020; van Schaik et al., 2020). Ganin et al. (2020) found that most organizations have a weak risk management foundation or lack appropriate processes, with much of the weakness attributed to the fast-changing nature of cybersecurity. However, there is value in exploring which cognitive biases and heuristics may contribute most to the deficiency (Kahneman et al., 2021; Rastogi et al., 2022).
Study Purpose
This qualitative exploratory study aimed to identify the cognitive biases in human decision-making most exhibited by cybersecurity professionals when providing risk ratings of potential third-party vendors. Fundamental concepts of decision theory, cognitive bias, and heuristics were used to frame and explore the decision-making process. The research methodology for the study was qualitative, using an interview-based data collection technique.
Qualitative methods were the most effective for collecting data about human decision-making (Ponto, 2015).
Research Question
The current qualitative exploratory study sought to identify the cognitive biases in human decision-making cybersecurity professionals exhibited when providing risk ratings of potential third-party vendors. Terrell (2022) explains that research questions should derive from the purpose statement, be clear and focused, be answerable through data analysis, have significance, and have a scope that can be handled in the time allotted. The research question met the characteristics outlined by Terrell. It was specifically worded to avoid bias or assumptions regarding the answer.
Q1
Which cognitive biases are most exhibited by cybersecurity professionals when providing risk ratings of potential third-party vendors?
Significance of the Study
The modern-day digital ecosystem is rife with security challenges that necessitate an accurate assessment of risks associated with third-party vendors (Berry, 2023; Boyson et al., 2021; Cybersecurity and Infrastructure Security Agency & National Institute of Standards and Technology, 2021; Eggers, 2021; Korolov, 2020; Kshetri, 2022; Pournader et al., 2020; Wolff et al., 2021). The current study extended a foundational idea from physics known as the observer effect, which posits that observation alters the observed phenomenon (Murphy, 2021). Analogously, awareness of the cognitive biases of cybersecurity professionals could prevent skewing risk assessment outcomes (Cains et al., 2022; Dror, 2020; White, 2023). By identifying and categorizing these biases, the study aimed to foster a transformation in how risk-based decisions are conceived and executed.
An extensive body of literature underscores the criticality of accurate risk assessment in cybersecurity, which is the bedrock for making informed decisions on vendor engagement (Fielder et al., 2018; Fleischman et al., 2023; Frietzsche, 2019; Joint Task Force Transformation Initiative, 2012; Wangen et al., 2017). Various scholars have spotlighted the human element as a potential weak link in cybersecurity, with cognitive biases being a significant concern (McAlaney & Benson, 2020). For instance, Tversky and Kahneman's (1974) seminal work on heuristics and biases explains how inherent biases could sway human judgment. A notion further extrapolated to the cybersecurity domain by contemporary researchers (Alnifie & Kim, 2023; Arellano et al., 2023; Berthet, 2021; Carmichael, 2020; Ceric & Holland, 2019; Dror, 2020; Johnson et al., 2020; Johnson et al., 2021; Kahneman et al., 2019; Korteling & Toet, 2022; Korteling et al., 2023; Monteiro et al., 2020; Rastogi et al., 2022; White, 2023; Yoon et al., 2021; Zhang et al., 2022).
Moreover, recent studies have explored the detrimental impact of cognitive biases on cybersecurity practices, emphasizing the necessity of objective risk assessment frameworks (McAlaney & Benson, 2020). Additionally, the literature suggests leveraging psychological principles to enhance cybersecurity measures, which aligns with the study's objective of applying the observer effect to understand and rectify biased risk assessments (Alnifie & Kim, 2023; Arellano et al., 2023; Berthet, 2021; Carmichael, 2020; Ceric & Holland, 2019; Dror, 2020; Johnson et al., 2020; Johnson et al., 2021; Kahneman et al., 2019; Korteling & Toet, 2022; Korteling et al., 2023; Monteiro et al., 2020; Rastogi et al., 2022; White, 2023; Yoon et al., 2021; Zhang et al., 2022).
The existing literature served as a platform to argue for the significance of the current research study. It is clear from the literature that cognitive biases challenge the objectivity of risk assessments in cybersecurity. The present study endeavored to unearth these biases and holds substantial promise in enhancing the risk assessment process. By spotlighting the deviations from objective, risk-based rationales in decision-making, the research could result in a paradigm shift related to how cybersecurity professionals approach risk assessments of third-party vendors.
Review of the Literature
Cognitive Neuroscience
Cognitive neuroscience is an interdisciplinary field that explores the underpinnings of neural processes and the role of the brain in mental functions (Li, 2023). The field blends the principles of psychology, which studies the mind and its processes, with those of neuroscience, which focuses on the nervous system. As a result, cognitive neuroscience seeks to understand how activities such as perception, memory, and decision-making are rooted in brain activity (Serra, 2021). Decision-making, as a complex cognitive function, has been an area of particular interest within cognitive neuroscience.
Cognitive neuroscience focuses on understanding the brain's role in decision-making by identifying specific neural pathways and brain regions activated during these processes (Li, 2023). Emotions, past experiences, biases, and external factors often shape our choices (Cains et al., 2022). By studying these neural underpinnings, researchers can gain insights into the reasons behind our decisions. Understanding the rationale behind decisions helps clarify how various internal and external elements influence our choices, providing a more profound comprehension of our decision-making mechanisms (Serra, 2021).
Within cybersecurity, strong decision-making and decision processes are paramount (Alecse, 2022). Whether it is a system administrator determining the best security protocols or a user deciding whether an email is trustworthy, continuous choices impact cybersecurity outcomes. Understanding the neural processes that drive these decisions can offer valuable insights (Alecse, 2022). For instance, recognizing that specific brain patterns correlate with risky decision-making might allow for better training or tools to help counteract these tendencies.
Just as software can have vulnerabilities, so too can the human mind. Cognitive neuroscience can unveil inherent biases or predispositions in decision-making that cyber-attackers might exploit (Cains et al., 2022). Phishing attacks, for example, often prey on cognitive biases by creating a sense of urgency or leveraging authority. Cybersecurity experts can develop more effective training programs and safeguards to protect against these psychological manipulations by understanding the neural basis of such biases (Cains et al., 2022).
In an age where cyber threats continue to evolve and become more sophisticated, integrating knowledge from cognitive neuroscience into cybersecurity strategies becomes critical (Andrade et al., 2022). Organizations can better equip their teams and users to recognize and respond to threats by understanding the brain's role in decision-making. Moreover, as cybersecurity increasingly incorporates artificial intelligence and machine learning, insights from cognitive neuroscience might inform the development of algorithms that mimic human decision-making processes, leading to more robust and adaptive security solutions (Naik et al., 2021).
Decision Theory
A key aspect of cognitive neuroscience is rooted in the theories behind how humans make decisions (Li, 2023). The discipline is not just a simple study of choice. It delves deeper into how and why certain decisions are made over others (Korteling et al., 2023). By understanding the factors and variables at play, decision theory aims to equip individuals and organizations with structured methods to arrive at optimal choices. The foundations of decision theory can be traced back to three key disciplines: probability, statistics, and economics (Serra, 2021).
Probability aids in understanding the uncertainties tied to decision-making, ensuring that choices are not just based on vague assumptions but on quantifiable risks (Serra, 2021). On the other hand, statistics allows for data analysis and past trends, giving decision-makers insights into potential outcomes and consequences of their choices (Serra, 2021). Finally, economics provides a framework for understanding the value and cost of decisions, ensuring that resources are allocated efficiently (Serra, 2021). Decision theory offers a comprehensive approach to decision-making by synthesizing principles from these three domains.
Decision theory acknowledges that decisions are often made in uncertain environments, with incomplete information and competing objectives (Yoon et al., 2021). Thus, methodologies and tools are designed to navigate these challenges (Fischhoff & Broomell, 2020). Addressing decision-making challenges ensures that choices are informed and aligned with goals and objectives. Whether for individuals facing everyday choices or organizations charting their future, decision theory aims to guide decision-makers toward better, more informed outcomes (Fischhoff & Broomell, 2020; Yoon et al., 2021).
System 1 and System 2 Thinking
System 1 and System 2 thinking represent two distinct modes of decision-making processes in the human brain, a dual-process theory that has significantly influenced psychology, behavioral economics, and neuroeconomics (Kahneman, 2011; Li, 2023; Serra, 2021; Sharp et al., 2022). System 1 is often described as the brain's fast, automatic, intuitive approach, requiring little effort and no sense of voluntary control (Kahneman, 2011). For example, when one instantly recognizes a face in a crowd or understands simple sentences in one's native language, System 1 is at work. Conversely, System 2 is characterized by slower, more deliberate, and more effortful processing, requiring conscious thought and attention (Kahneman, 2011). Solving a complex mathematical problem or deciding after careful consideration engages System 2 processes. System 2 thinking is associated with the subjective experience of agency, choice, and concentration.
The interplay between these two systems is crucial for understanding human behavior, especially in decision-making and judgment (Fischhoff & Broomell, 2020; Kahneman & Frederick, 2005; Kahneman et al., 2021; Korteling & Toet, 2022; Korteling et al., 2023; Milkman et al., 2009). System 1 can lead to biases and heuristics that influence judgments and decisions in ways that are not always rational or optimal (Berthet, 2021). System 2, while capable of correcting these biases, often relies on System 1 for initial impressions and judgments, which it may or may not override (Carmichael, 2020). Critics of the dual-process theory argue that the distinction between System 1 and System 2 may be too simplistic and that the processes are more interconnected than previously thought (Ketchen Jr. & Craighead, 2022). Recent neuroeconomics and cognitive neuroscience research continues to explore the complex relationship between these two systems, suggesting that decision-making involves a dynamic interaction between automatic and controlled processes rather than a simple dichotomy (Korteling & Toet, 2022; Li, 2023; Serra, 2021; Sharp et al., 2022).
Understanding System 1 and System 2 thinking provides insight into human cognition and behavior and offers practical implications for various domains, including marketing, policy-making, and personal decision-making (Cox, 2023). By recognizing the influence of automatic, intuitive thinking, individuals and institutions can design better interventions and strategies to improve decision outcomes and behavioral patterns (Arellano et al., 2023). Exploring System 1 and System 2 thinking opens new avenues for research and application regarding the complexities of human decision-making (Panda, 2022). The existing literature emphasizes the need for a nuanced approach to analyzing behavior that accounts for both the automaticity of human cognition and the deliberative processes that can override or modify instinctual responses.
Cognitive Bias
Cognitive biases, deeply ingrained in human psychology, significantly influence our decision-making processes (Berthet, 2021; Ceric & Holland, 2019; Deniz, 2020; Dror, 2020; Kahneman et al., 2019; Korteling et al., 2023). Defined succinctly, cognitive bias is a systematic pattern of deviation from norm or rationality in judgment (Dror, 2020). These biases often divert individuals from logical or normative reasoning, leading them to make judgments that may not always align with objective reality. Pioneers in the study of these biases, Amos Tversky and Daniel Kahneman (1974), made groundbreaking contributions to the understanding of this phenomenon. Their seminal work highlighted humans' frequent deviations from statistical reasoning when faced with decisions ). Through a series of innovative experiments, they demonstrated that individuals often resort to heuristics or mental shortcuts. While these heuristics can be efficient, they can also result in systematic errors or biases.
The availability bias is one of the most notable biases they identified (Tversky & Kahneman, 1974). Availability bias causes individuals to estimate the likelihood of an event based on how easily examples come to mind (Korteling & Toet, 2022). However, this often leads to miscalculations of actual probabilities, as the most memorable events are not always the most common. Tversky and Kahneman's research illuminated various cognitive biases, such as anchoring, which is influenced by initial information when making subsequent judgments (Tversky & Kahneman, 1974). Another bias they identified is confirmation bias, where individuals tend to favor information that aligns with their pre-existing beliefs (Korteling & Toet, 2022). They also highlighted the representativeness heuristic, where people assess probabilities by comparing events to known prototypes (Tversky & Kahneman, 1974).
While Tversky and Kahneman laid the foundation for understanding these biases, exploring this subject did not end with them. The works of Berthet, Ceric, Deniz, Dror, Ganin, and many others have expanded on this foundational knowledge, each contributing unique insights and perspectives (Berthet, 2021; Ceric & Holland, 2019; Deniz, 2020; Dror, 2020; Ganin et al., 2020). Their pioneering work acted as a catalyst, inspiring much subsequent research. Scholars and researchers delved deeper into the intricate manifestations of cognitive biases, seeking to understand their origins, impacts, and potential strategies for mitigation.
In recent years, the study of cognitive biases has taken a new turn with the integration of emerging technologies and the rise of big data (Alghamdi & Al-Baity, 2022; Belhadi et al., 2021; Booch et al., 2021; Deiva Ganesh & Kalpana, 2022; Lee & Mangalaraj, 2022; Maheshwari et al., 2020; Naik et al., 2021; Toorajipour et al., 2021). These advancements offer fresh avenues to dissect and comprehend the intricate interplay of cognitive biases in human decision-making. Researchers like Balayn, Cox, Jarjoui, Lee, and Mitchell have explored how technology can both exacerbate and mitigate the effects of these biases (Balayn et al., 2021; Cox, 2023; Jarjoui & Murimi, 2021; Lee & Mangalaraj, 2022; Mitchell, 2022). For instance, while algorithms can perpetuate biases present in their training data, they can also be designed to identify and correct for these biases, offering a more objective perspective (Baer, 2019; Binns, 2022; Burton et al., 2019; Creel & Hellman, 2022; Cucu et al., 2019; Miles, 2021).
Anchoring bias is a particularly intriguing cognitive pitfall where individuals heavily rely on the first piece of information they encounter (the "anchor") when making decisions (Johnson et al., 2020). For instance, when negotiating a price, the first number mentioned often becomes the reference point, influencing subsequent discussions regardless of its relevance. Pricing bias can lead to suboptimal decisions, as individuals may not adjust sufficiently away from this anchor, even when presented with more accurate or relevant information.
Availability bias and confirmation bias often work hand-in-hand. The former refers to the tendency of individuals to base their judgments on readily available or recent information, often overlooking comprehensive data (Johnson et al., 2020). The first biases can lead to skewed perceptions, especially in a world saturated with information where the most dramatic or sensational events are remembered. On the other hand, confirmation bias is the inclination to seek, interpret, and remember information in a way that confirms one's pre-existing beliefs (Johnson et al., 2020). The second bias can create a feedback loop, where individuals become more entrenched in their views, disregarding evidence to the contrary. The Sunk Cost Bias further complicates decision-making, as individuals continue a behavior or endeavor based on previously invested resources (time, money, or effort), even if it is no longer beneficial (Johnson et al., 2020). Sunk cost bias can lead to irrational decisions, as past investments should not influence current choices.
Several other biases also play pivotal roles in shaping human judgment. The Peltzman Effect describes where people might take more significant risks when they perceive increased safety measures (White, 2023). Anecdotal evidence bias makes individuals prioritize personal experiences or isolated examples over extensive data or statistical evidence (Fleischman et al., 2023). Omission bias judges harmful actions worse than equally harmful inactions (Berthet, 2021). Overconfidence bias makes individuals overestimate their knowledge or abilities while framing bias refers to being influenced by the way information is presented rather than the information itself (Berthet, 2021; Zhang et al., 2022). Optimism bias leads people to believe they are less likely to experience adverse events compared to others (Alnifie & Kim, 2023). Lastly, the self-serving bias is the habit of attributing positive events to one's character but attributing adverse events to external factors (Carmichael, 2020). These biases paint a picture of the intricate web of cognitive shortcuts and pitfalls that influence human decision-making, emphasizing the importance of awareness and critical thinking in our daily choices.
Heuristics
Heuristics, often called mental shortcuts, are humans' innate cognitive tools to simplify daily decisions (Andrade et al., 2022; Simon, 1955). These strategies have evolved, allowing our ancestors to make quick judgments in environments where rapid decision-making was crucial for survival (Fischhoff & Broomell, 2020). In the modern world, heuristics help individuals cut through the overwhelming influx of information, enabling them to process and react without being paralyzed by analysis. By reducing the cognitive load, heuristics allow the brain to function more efficiently, reserving its resources for other essential tasks.
However, the use of heuristics is not without its pitfalls. While they expedite decision-making, they can also introduce cognitive biases, leading individuals astray from objective or rational choices (Fischhoff, 2003). A classic example is the representativeness heuristic, where individuals assess probabilities based on how much a situation resembles a prototype in their minds (White, 2023). While this can be useful in some scenarios, it often results in overconfidence, causing individuals to overlook other crucial factors. Another heuristic, the availability heuristic, influences judgments based on the ease with which examples or memories can be recalled (White, 2023). Availability bias can lead to skewed perceptions, especially in a media-saturated world where dramatic or recent events are more easily remembered than statistical norms.
The intricate relationship between heuristics and biases underscores a fundamental aspect of human cognition: the trade-off between efficiency and accuracy (M'Manga, 2020). On one hand, heuristics enable the brain to process information swiftly, ensuring timely reactions. On the other hand, this speed can come at the cost of precision, leading to potential errors in judgment. Recognizing this delicate balance is essential, as it shapes the foundation of human cognitive architecture (M'Manga, 2020).
Understanding the dynamics of heuristics and cognitive biases has practical implications. By delving deeper into these cognitive processes, individuals and institutions can strategize to harness the benefits of heuristics while minimizing the pitfalls of biases (Adebayo, 2022; Dror, 2020). Creating a decision-making strategy involves cultivating an environment where intuitive, heuristic-driven thinking coexists harmoniously with analytical and deliberate reasoning. By achieving this equilibrium, organizations can foster a culture of informed decision-making, ensuring they are better equipped to navigate the complexities of our information-dense world (Arellano et al., 2023). The journey to understand and leverage heuristics while being wary of biases is a testament to the human endeavor to optimize cognition in an ever-evolving landscape.
Cyber Supply Chain Risk Management
Supply chain risk management (SCRM) is crucial in ensuring the security and resilience of organizations, as it helps to identify, assess, and mitigate risks associated with the supply chain. The growing interconnectivity and complexity of supply chains make SCRM a critical component in protecting against various threats, including cyber-attacks, natural disasters, and human errors (National Counterintelligence and Security Center, 2020). According to CISA, SCRM helps organizations maintain the confidentiality, integrity, and availability of their systems and information and ensures the continuity of operations (Cybersecurity and Infrastructure Security Agency & National Institute of Standards and Technology, 2021). Effective SCRM practices also help organizations make informed decisions, reduce costs associated with supply chain disruptions, and enhance their overall security posture.
Cyber Supply Chain Risk Management (C-SCRM) is critical in managing a supply chain's security, resilience, and integrity, which is often multifaceted and interconnected (Exec. Order No. 14017, 2021). The cyber supply chain refers to the ecosystem of organizations, people, activities, information, and resources in creating and delivering digital products and services (National Counterintelligence and Security Center, 2020). The supply chain elements include software developers, hardware manufacturers, and service providers (National Counterintelligence and Security Center, 2020). C-SCRM seeks to assess and mitigate the vulnerabilities and threats across the supply chain that could impact the confidentiality, integrity, and availability of information and information systems. The risk management process involves identifying the various entities in the supply chain, understanding the flow of information and resources, and assessing the risks associated with each entity and their interconnections.
One fundamental aspect of C-SCRM is identifying risks arising from dependencies and vulnerabilities within the supply chain, such as those due to the integration of insecure components or services or from suppliers with inadequate security practices (Melnyk et al., 2022). After identifying such risks, organizations must employ strategies to manage and mitigate them. Strategies can involve implementing security controls, enhancing contractual clauses and procurement processes, and continuously monitoring the security posture of suppliers (Elangovan, 2019). C-SCRM is particularly crucial as supply chains become more global and interconnected and as reliance on third-party products and services increases (Latif et al., 2021). A compromise in any part of the supply chain can have cascading effects, impacting numerous entities up and down the supply chain. For instance, a vulnerability in a software component can affect all products and services that utilize that component, potentially leading to widespread data breaches, system outages, or other adverse impacts (Wolff et al., 2021). Effective cyber supply chain risk management requires a holistic and proactive approach, combining organizational processes, technology solutions, and human factors. It necessitates collaboration, information sharing among supply chain entities, and a shared responsibility for managing risks.
Gaps in the Literature
Most research on decision-making, particularly risk-based decision-making, was centered on industries other than cybersecurity. The lack of focus was a significant oversight, especially considering the increasing importance of cybersecurity in today's digital age. While finance, healthcare, and manufacturing industries have been studied in risk assessment and decision-making processes, cybersecurity remains relatively underexplored (Goel et al., 2020; Kahneman et al., 2021; Reed, 2020). The gap in research was particularly glaring given the unique challenges posed by cybersecurity threats, which often differ in nature and complexity from risks in other sectors (Singh et al., 2023).
As the digital landscape continues to evolve, so does the nature of threats and vulnerabilities. Cybersecurity is not just an IT concern but a strategic imperative for organizations across all sectors (Snow, 2020). My field and concentration are squarely focused on understanding the nuances of decision-making processes in cybersecurity. By not adequately addressing this area, the literature failed to provide insights directly applicable to one of the most pressing challenges of our time.
When the literature touched on cybersecurity risk decision-making, it predominantly zeroed in on internal, first-party decisions or the organization's role within the supply chain (Cheung et al., 2021; Colicchia et al., 2019; Melnyk et al., 2022; Sawik, 2022). The narrow scope of the literature overlooked the broader ecosystem in which organizations operate. In today's interconnected world, an organization's cybersecurity posture is influenced by more than internal decisions. It is also influenced by the decisions of its partners, suppliers, and even customers.
While there was some acknowledgment of the importance of supply chain risk in the context of cybersecurity, most research stops at merely establishing its significance (Cheung et al., 2021; Colicchia et al., 2019; Melnyk et al., 2022; Sawik, 2022). Recent breaches have underscored the need for a deeper dive into this area, yet the literature largely remains on the surface (Eggers, 2021; Wolff et al., 2021). There was a pressing need for comprehensive studies highlighting the importance of supply chain risk and providing actionable insights and frameworks for addressing it (Boyson et al., 2021).
Of the gaps identified, the most significant was the superficial treatment of supply chain risk. Addressing this gap was crucial because supply chain vulnerabilities can serve as entry points for cyber adversaries, potentially compromising one organization and several entities linked through the supply chain. By delving deeper into supply chain risk, we can better understand the intricacies of this challenge and develop more robust strategies to address it (Colicchia et al., 2019; Melnyk et al., 2022). A more thorough understanding enhances the cybersecurity posture of individual organizations and strengthens the broader business ecosystem. Addressing this gap informed the problem by providing organizations with the tools and knowledge to safeguard themselves and, by extension, their partners from cyber threats.
Methodology, Design, and Methods
Conceptually, the research focused on cyber-risk decision-making. It explored the divergence between the theoretical underpinnings of decision-making and the practical manifestation that demotions often influence decision-making. By identifying the most common cognitive biases in cyber-risk assessments, the research aimed to provide actionable insights to organizations, enabling more effective risk management strategies for vendor selection and cybersecurity in general.
Research Methodology and Design
For the past three decades, quantitative research methods have been the general research approach (Hitchcox, 2020). However, much of the contemporary research conducted in the cybersecurity industry is exploratory and nascent (Hitchcox, 2020). Additionally, the primary objective of qualitative research is to understand the experiences of an individual, which is the central goal of the proposed research question. Lastly, a qualitative methodology illuminates the rationale of human decision-making and perceptions (Hitchcox, 2020).
Most cyber risk management models are labeled quantitative. However, upon further examination, the models are found to be, in reality, qualitative (Snow, 2020). The rationale for the conclusion that most cybersecurity risk management models are qualitative is that even quantitative models depend on human interaction (Hitchcox, 2020). Human interaction, by definition, also involves judgment and decision-making (Hitchcox, 2020). The study examined specific decisions by extending the existing decision-making theory. Study participants' responses to the questions of motivation and rationale for their decisions provided clues as to which bias or heuristics were most prevalent.
In addition, the interpretive paradigm emphasizes the role of context in shaping human behavior and decision-making (Kadyschuk, 2023). Establishing context is particularly relevant in studying cognitive biases and risk-based decision-making, as situational factors and social context influence these phenomena. The interpretive paradigm encourages researchers to examine the social and cultural factors that shape decision-making processes and to consider the subjective experiences and interpretations of individuals involved in these processes.
Population
According to the ISC2 (2023), the number of cybersecurity professionals in the global workforce was estimated at 5.5 million, with 1.5 million in the US. A search of people on LinkedIn using the term "cybersecurity" and limiting the location to the United States, resulted in a total population of about 586,000 people. The target population was also highly specialized and not identifiable based on job titles or basic demographic information. Specific criteria were outlined for the solicitation of study participants. The requirements included a statement that the individual should have experience with cybersecurity risk assessment of third parties and not be at a Chief Information Security Officer (CISO) or equivalent level. Because of the varied structure of vendor risk management teams at organizations, there was no expectation or criteria requiring a threshold percentage of current job responsibilities related to cybersecurity risk assessment of third parties.
Sample
The qualitative design of the study, along with the target population's specialized characteristics, justified using a non-probability purposive sample as both acceptable and appropriate (Bamberger & Mabry, 2020). Employing this method, I ensured that the participants selected were likely to provide rich, relevant, and insightful data crucial for the depth and validity required by qualitative research. The selection approach allowed for a focused study, delving deeply into the experiences and perspectives of a targeted group, which was essential for the nuanced understanding of the specific phenomenon under investigation. The sampling method was particularly fitting for studies exploring a phenomenon within a specific group (Bamberger & Mabry, 2020). The estimated sample size was expected to be between 8 and 10 participants (Hennink & Kaiser, 2022).
Findings
The qualitative research interviews presented three vendor scenarios and the same three questions for each scenario. The specific scenarios presented to study participants have been included in the Appendix . Each interview lasted approximately 20 minutes. All participants were recruited using LinkedIn, and all interviews were conducted using Zoom.
The scenarios and follow-up questions were presented in the same order, and the wording was not modified for participants. After each scenario, participants were asked how they would rate the risk of engaging with the vendor described. The participants were then asked to explain their rationale for evaluating the vendor's risk. Lastly, the participants were asked how confident they were in the rating they provided.
Table 2 documents the key codes utilized in the analysis, providing clear definitions and explanations. The documentation acts as a reference guide, much like a dictionary, ensuring that researchers have a shared understanding of how to categorize and interpret data consistently. It establishes a common language for data analysis, making it easier for future researchers to follow the same coding process and maintain the integrity of the research.
Major Theme 1: Data-Driven Decision-Making (Frequency: 18)
The study results indicated that cybersecurity risk management professionals tend to become more biased as they gain experience evaluating third-party vendors. The progression of bias can be attributed to the increased reliance on intuitive judgments and past experiences, a hallmark of System 1 thinking (Cox, 2023). With experience, professionals might develop a sense of overconfidence in their ability to evaluate vendors based on gut feelings or familiarity (Cains et al., 2022). Overconfidence can lead to a reduced emphasis on analytical and methodical evaluation, typically associated with System 2 thinking (Cox, 2023). As a result, experienced professionals might overlook new or subtle risks vendors present, assuming that their past experiences are sufficient for accurate assessments.
The emphasis on data-driven decision-making exhibited by the more inexperienced study participants reflected a less heuristic approach to risk assessment. These professionals had not yet established mental shortcuts or routines that allowed for shortcutting the assessment process (Gigerenzer, 2023). As a result, they took a more academic approach to evaluating the sensitivity of shared data, compliance with industry regulations, and the vendor's data handling practices. This approach lets organizations objectively measure and compare the risks associated with different vendors, facilitating more informed decision-making.
As cybersecurity professionals accumulate experience, there was an observable shift towards more intuitive, heuristic-based decision-making (Cains et al., 2022; Dror, 2020; White, 2023). Such reliance on heuristics can lead to systematic errors in judgment, as these shortcuts are often influenced more by personal experiences and less by factual, unbiased analysis (Cains et al., 2022). A shift toward more heuristic thinking also results in an increased reliance on System 1 thinking, characterized by fast, automatic responses, at the expense of the slower, more deliberate System 2 thinking (Cox, 2023). The change in cognitive processing leads to a higher propensity for biased evaluations, as professionals lean more on their internal biases and less on objective, analytical assessments of third-party vendor analysis (Cains et al., 2022). The experience trend underscores the need for continuous training and awareness of cognitive biases, even among seasoned professionals, to ensure robust and unbiased cybersecurity risk assessments.
Major Theme 2: Authority Bias (Frequency: 18)
Authority bias in the fictitious scenarios used in the study manifested as deference to external validations, such as industry certifications or endorsements from trusted authorities. A reliance on authority can streamline the decision-making process by allowing the assessor to use these certifications as shortcuts to establish trustworthiness without conducting in-depth analyses for every vendor (Korteling & Toet, 2022). However, this bias may also lead to overreliance on such credentials, potentially overlooking other critical risk factors not covered by certifications. It suggests that while certifications are valuable, they should not be the sole criterion for vendor selection, emphasizing the importance of a holistic risk assessment approach.
In the context of third-party risk assessment, overreliance on external audits and certifications can present significant risks. The Payment Card Industry Data Security Standard (PCI-DSS) is an international information security standard that aims to protect credit card data and sensitive authentication data and reduce credit card fraud (Lincke, 2024). It applies to all organizations that process credit card data, especially those in the heavily regulated finance industry. SOC2 Type 2 reports comprehensively evaluate a company's data management practices over time, focusing on security, availability, processing integrity, confidentiality, and privacy (Sabillon, 2022). However, they reflect the company's controls during the audit period and do not guarantee future compliance or effectiveness. ISO27001 certification assesses the establishment and maintenance of an information security management system. ISO27001 emphasizes procedural correctness rather than the effectiveness of these processes in real-world scenarios (Sabillon, 2022).
The downside of authority bias lies in its potential to stifle critical examination (Einhorn, 2023). Organizations might overlook emerging vendors who offer innovative solutions but lack the established credentials of their more established counterparts. The discounting of certain vendors could result in missed opportunities for adopting more effective or efficient technologies (Einhorn, 2023). Balancing respect for authoritative endorsements with critically analyzing all relevant risk factors is crucial for making well-rounded vendor selection decisions.
An overreliance on audits and certifications can create a deceptive sense of security. These assessments, while valuable, are snapshots in time and do not continuously account for emerging security challenges. Furthermore, they cannot guarantee that their evaluation controls will remain effective beyond the evaluation period (Culot et al., 2021). A lack of assurance can lead to vulnerabilities that malicious actors may exploit. Therefore, it is crucial to maintain ongoing vigilance and adaptation in third-party risk management. A robust approach ensures that security measures evolve with the threat landscape, protecting what periodic assessments offer.
Major Theme 3: Ambiguity Bias (Frequency: 17)
The study revealed a notable paradox in the approach to decision-making. Cybersecurity professionals demonstrated a strong dislike for ambiguity, preferring scenarios with clear and detailed information. The preference for clarity was evident in participants' responses to the ambiguous elements of the scenarios in the study. Participants tended to assess vendors as having a higher risk due to the lack of detailed information. Considering the inherent ambiguity in cybersecurity, an overwhelming desire for clarity was particularly paradoxical.
The paradox lies in the fact that while cybersecurity professionals seek to minimize uncertainty in their assessments and strategies, they operate in a field where uncertainty is a constant (Singh et al., 2023). Cybersecurity demands high adaptability and a tolerance for ambiguity, traits that might not come naturally to those who prefer clear and structured information. Cybersecurity professionals' aversion to ambiguity could limit their effectiveness in a field requiring flexibility and navigating unknowns (Zhang et al., 2022).
The ambiguity paradox highlighted a broader challenge in cybersecurity risk management: the need to balance the desire for data-driven, certain decisions with the practical realities of an ever-changing threat landscape (Santini et al., 2019). The paradox becomes even more pronounced when assessing third-party vendors. In these situations, control and transparency are often more limited, exacerbating the challenges for cybersecurity professionals (Sawik, 2022). Risk Assessors must rely on vendor assurances, external audits, and certifications, which may not fully align with their desire for clear, detailed information about security practices and risk exposures. The ambiguity paradox suggests that cybersecurity professionals may need to develop strategies and tools to manage ambiguity better, developing an ability to respond to unforeseen threats and adapt to new information (Mitchell, 2022).
Major Theme 4: Bandwagon Bias (Frequency: 9)
Bandwagon bias demonstrated how the popularity of a vendor within an industry or peer group can influence decision-making, sometimes leading organizations to choose vendors based more on their widespread acceptance rather than an independent assessment of their suitability. Reliance on the mass use of a vendor can create a sense of security in numbers, as decision-makers assume that the collective wisdom of their peers equates to a safe choice. However, bandwagon bias may discourage critical evaluation and the consideration of less popular, albeit potentially more suitable, alternatives (Korteling et al., 2023).
In the first scenario, the emphasis on the vendor's previous experience with similar large-scale organizations, as highlighted by Participant 003, reflected a tendency to trust a vendor more if it has serviced other entities, suggesting that the vendor's worth was measured by its association with other successful companies. The second scenario further reinforced the trust of vendors, where Participant 003 and Participant 006 commended the vendor for managing large transactions and having an established reputation, indicating a predisposition to equate past successes with future reliability. The mention by Participant 006 about the importance of understanding the risks associated with a widely used vendor underscored a herd mentality, where the vendor's popularity among competitors is seen as a hallmark of reliability despite potential risks. Participant 008's concern about competitors leveraging predictive analytics and Participant 009's note on a competitor's successful use of the vendor during losses suggested that the decision-making was influenced by competitors' actions rather than an independent assessment of the vendor's capabilities. Lastly, Participant 010's commendation of the vendor for its strong customer base and seamless processing, despite regulatory challenges, showcased a reliance on the vendor's market presence as a proxy for quality and reliability, further evidencing how bandwagon bias can shape perceptions and decisions in business contexts.
As previously discussed, the risk with bandwagon bias is the potential for a 'herd mentality,' where decisions are made based on trends rather than tailored analysis of an organization's specific needs (Huo et al., 2020). While following industry trends can provide valuable insights, organizations must remain vigilant in independently assessing each vendor's risks and benefits. Diversifying sources of information and encouraging a culture of skepticism can help counteract the bandwagon effect, ensuring that decisions are made based on a comprehensive understanding of available options (Huo et al., 2020).
Major Theme 5: Familiarity Bias (Frequency: 6)
Familiarity bias, also known as the mere exposure effect, is a cognitive bias where individuals tend to develop a preference for things simply because they are familiar with them (Korteling & Toet, 2022). People often focus on information they are already familiar with when making decisions, which can lead to irrational decision-making. Familiarity bias arises from cognitive ease, where familiar options are processed more easily and appear more appealing (Berthet, 2021). A bias towards the familiar can simplify decision-making and minimize perceived risk, as there is a track record of performance to refer to. However, it may also hinder the evaluation of new vendors who could offer more innovative or cost-effective solutions (Korteling & Toet, 2022).
In Scenario 2, the manifestation of familiarity bias was evident as participants strongly preferred an established service provider with whom their organization had a long-standing and reliable partnership. The preference for an established service provider was highlighted by Participant 001's acknowledgment of the perceived risk but still favoring the provider due to over a decade of reliable partnership. Similarly, Participant 006 valued the vendor's experience handling large transactions, reinforcing the trust in the provider's capabilities. Participants 007 and 008 further emphasized the significance of a trusted relationship, with Participant 008 specifically naming PaySphere as a reliable partner for over a decade. Scenario 2 demonstrated how familiarity bias influences decision-making, where the comfort and trust built from a prolonged partnership outweighed potential risks or considerations of alternative options.
Familiarity bias can lead to a reluctance to switch vendors, even when it might be in an organization's best interest to do so (Sumera & Reddy, 2020). It underscores the importance of maintaining an open-minded approach to vendor selection, where past experiences inform but do not dictate future decisions. Encouraging periodic reviews of vendor relationships and market offerings can help mitigate the impact of familiarity bias, ensuring that organizations are always aligned with the best available options (Sumera & Reddy, 2020).
Major Theme 6: Anti-Innovation Bias (Frequency: 5)
Anti-innovation bias reflects a cautious approach to adopting new technologies, where the perceived risks of innovation outweigh the potential benefits or where ambiguity is overwhelming (Korteling & Toet, 2022). The more that is unknown about an innovation, the more bias there is against it. Skepticism towards innovation can be prudent, helping to avoid untested technologies that may not provide secure or reliable solutions (Vereschak et al., 2021). However, it can also prevent organizations from gaining a competitive edge through early adoption of breakthrough technologies.
In Scenario 3, participants' diverse perspectives on artificial intelligence (AI) illustrated the complex nature of anti-innovation bias. Participant 002 highlighted a mixed bias towards AI, indicating skepticism and optimism. Participant 004 pointed out AI's cutting-edge status and acknowledged a general lack of understanding about its intricacies, suggesting a barrier to acceptance due to unfamiliarity. Concerns about security in a Software as a Service (SaaS) context and the need for cautious implementation were raised by Participant 005, emphasizing the perceived risks associated with adopting new technologies. Additionally, Participant 007's lack of confidence in AI vendors' reliability underscored a mistrust in the stability and dependability of emerging innovations. These viewpoints reflected a multifaceted anti-innovation bias rooted in skepticism, fear of the unknown, security concerns, and mistrust towards new technological advancements.
Overcoming anti-innovation bias requires a balanced approach that recognizes the value of innovation while acknowledging its risks (Enders et al., 2020). Organizations can foster an environment that encourages the exploration of new technologies within a framework of rigorous risk assessment. By conducting pilot projects or phased rollouts for innovative solutions, organizations can assess their impact and integration challenges in a controlled manner (Enders et al., 2020). Pilots and proofs of concept allow for the careful evaluation of the benefits of new technology while considering the potential risks, thus enabling a more informed approach to innovation.
Less Frequent Biases
The present study of cognitive biases in cybersecurity risk decision-making uncovered a nuanced landscape where even biases that occur less frequently when aggregated significantly influence outcomes. Despite these biases appearing less frequently than previously, their cumulative effect became evident through the analysis of participant responses. The accumulation of biases highlighted the complexity and subtlety of how cognitive bias impacts risk assessment and decision-making processes.
In the study results, anchoring bias was less common when examined independently. However, a few participants expressed that they relied on the information presented in the scenario to make judgments, revealing anchoring bias's subtle yet significant influence. Moreover, anecdotal evidence bias emerged as another factor, with participants shaping their risk assessments based on the experiences of others rather than an objective review of the scenario. The Peltzman Effect, although less frequently observed, demonstrated how perceived safety measures could unconsciously lead assessors to justify riskier behaviors, further complicating accurate risk assessment.
Lastly, the self-serving bias, overgeneralization, conjunction bias, and pricing bias, though not as frequent as other biases in this study, contributed to a complex fabric of decision-making rationale that became notable when aggregated. For example, the tendency to want to avoid being viewed as responsible for a data breach (self-serving bias) or to make generalizations based on a single piece of information (overgeneralization and conjunction bias) showcases how these biases, though individually infrequent, collectively led to subjective risk assessments. Pricing bias further complicated the landscape, with a participant explaining that they leveraged cost concerns as a primary rationale for their assessment.
Aggregating these less frequent biases underscored a critical insight into cybersecurity risk decision-making: the collective impact of biases, even sporadically, can significantly distort risk assessments and decisions. Recognizing the compounding nature of cognitive bias highlights the importance of comprehensive strategies to mitigate the influence of biases. By acknowledging and addressing the cumulative effect of both major and less frequent biases, organizations can foster more informed, rational, and effective cybersecurity risk management practices (Arellano et al., 2023). Self-awareness and mitigation of bias enhance the accuracy of risk assessments and strengthen the overall decision-making framework by ensuring that even the subtlest biases are recognized and countered, leading to more robust and resilient cybersecurity strategies.
Practice Implications of Study Findings
Expanding on the themes in this study reveals a nuanced landscape of decision-making in vendor risk assessment. While data-driven decision-making provides a solid foundation for objective analysis, the influence of psychological biases illustrates the complexity of human judgment in corporate environments. Leveraging structured processes, continuous education, and a culture that values security and innovation can help organizations make more informed, balanced decisions. An objective, repeatable approach enhances risk management practices and supports strategic agility in navigating the evolving vendor landscape.
Implication 1
The current research study's assertion that cognitive bias precipitates suboptimal decision-making was the cornerstone. A cognitive bias essentially signifies a systematic pattern of deviation from norm or rationality in judgment, where individuals create their own "subjective reality" from their perception (Korteling et al., 2023). Examining these biases was crucial as they can obfuscate the factual assessment of risks and, thus, lead to misguided decisions (Arellano et al., 2023). Through thorough analysis, the study worked to unearth how cognitive biases affect the decision-making processes inherent in third-party risk management. The current research study was a step toward developing more robust risk management strategies.
Implication 2
The quest to understand and mitigate the detrimental effects of cognitive biases is not a mere academic exercise but a necessity for fostering a more secure digital supply chain ecosystem. The study aimed to create a nexus between cognitive science and cyber supply chain risk management. Leveraging and enhancing the current research will naturally yield a more holistic understanding of the challenges. The outcome will be a more secure, resilient, and efficient digital supply chain. By bridging the gap between these domains, the study created a robust foundation for future research and practical initiatives to enhance the security and efficiency of digital supply chains amidst an ever-evolving cyber threat landscape.
The research revealed the most prevalent cognitive biases in assessing third-party vendor cybersecurity risk. Identifying and acknowledging the role of these biases in decision-making helps improve cyber risk professionals. With the most prevalent biases identified, cyber risk professionals can consciously change how they evaluate threats and dangers. A change in approach and understanding will make assessing risks more effective and accurate. The results from this study can also be transferred to other areas of cyber risk beyond third-party risk management. The transferability can improve all areas of risk evaluation.
Implication 3
One of the practical benefits of addressing the cognitive biases in third-party risk assessment is the potential to improve the well-being and performance of the cybersecurity professionals themselves. A more objective and consistent process of evaluating vendors will reduce the cognitive load and emotional stress often accompanying heuristic-based judgments (Dror, 2020). It will also promote a more collaborative and equitable work environment, where the opinions and expertise of different team members are valued and respected rather than being overshadowed by a few dominant or experienced voices (Cox, 2023). Furthermore, it will enhance the quality and reliability of the assessment ratings, as they will be less prone to unwanted variation and subjective interpretation (Cains et al., 2022). These improvements will benefit cybersecurity professionals, organizations, and stakeholders that rely on their assessments.
Recommendations for Further Research
There are several areas of research that I would like to explore, as well as ample areas of research for others to build on. First, as mentioned earlier, it would be illuminating to investigate the extent to which CISOs and CISO equivalents can mitigate the biases of those who report to them. At the same time, it would be interesting to see what biases these senior leaders introduce because of the heuristics they leverage as busy executives. Based on the impact of the experience of the study participants on the current study, I would be curious to explore what previously unexhibited cognitive biases are displayed by leaders who are the most heuristically driven in an organization.
Another recommendation for future research is to add more vendor risk scenarios and randomize the order in which the scenarios are presented to study participants. The benefit of adding more scenarios would be more opportunities to exhibit cognitive biases, allowing for validation that the participant favors a specific bias. Randomizing the order of the scenarios will also allow for examining the role that anchoring and framing play in risk assessments. Glimmers of anchoring and framing were seen in the current study. A more deliberate examination of these concepts would be a worthy endeavor.
The emergence of advanced technologies, including artificial intelligence, presents a promising enhancement for unraveling the complex interplay of biases and heuristics. Researchers can explore how these cognitive phenomena manifest and interact in real-world scenarios through data analytics and cognitive modeling. Furthermore, technology can serve as a tool for individuals and organizations to identify and mitigate biases and irrational heuristics, fostering a culture of rational decision-making. As technological advancements continue, the potential to address the biases and heuristics in human cognition increases, paving the way for more rational and data-driven decision-making.
Lastly, a study leveraging a quantitative methodology would allow future researchers to examine the most prevalent cognitive biases and the intensity of the impact created by the biases. A more statistical approach would provide a more detailed and nuanced understanding of the role of cognitive biases in third-party risk assessment. By quantifying the impact of these biases, researchers could develop more targeted and effective interventions to mitigate their effects. A quantitative methodology would also allow statistical analysis to identify patterns and relationships between different variables, providing a more robust and comprehensive understanding of the factors influencing third-party risk assessment.
Conclusion
The study's qualitative nature, focusing on interviews and thematic analysis, identified key biases such as authority bias, ambiguity aversion, and experience-induced bias. These biases affect the accuracy and reliability of risk assessments by leading professionals to over-rely on external certifications, avoid ambiguous information, and rely too heavily on past experiences. The findings suggested that these cognitive biases impact the effectiveness of cybersecurity risk management. The study emphasized the importance of addressing these biases to improve decision-making processes, suggesting that more objective and consistent evaluation methods could enhance the cybersecurity field's effectiveness and resilience.
The study underlined the need for a deeper investigation into how senior executives can actively counteract cognitive biases in cybersecurity risk assessments. It suggested that understanding the influence of leadership could unveil strategies to foster a more objective decision-making environment. Additionally, by exploring a broader array of risk scenarios, researchers can identify how biases manifest across different contexts, potentially uncovering industry-specific vulnerabilities. The adoption of quantitative methods is also recommended to accurately measure the impact of these biases, providing a more empirical foundation for developing interventions. Such research could pave the way for more robust risk management frameworks, enhancing the cybersecurity domain's capacity to deal with the evolving landscape of third-party vendor risks.
In cybersecurity, recognizing and mitigating cognitive biases in risk assessment is crucial for ensuring thorough and accurate evaluations. A proactive approach reduces vulnerabilities and strengthens digital supply chain security by promoting a culture of critical analysis and skepticism toward potentially biased judgments. For cybersecurity professionals, it involves adopting structured decision-making strategies that explicitly account for these biases, such as implementing checklists, conducting peer reviews, and utilizing decision-support tools. These measures help foster an environment where decisions are made based on data and objective analysis rather than intuition or flawed heuristics. By systematically addressing cognitive biases, organizations can improve their resilience against cyber threats, enhancing their ability to protect sensitive data and maintain trust in an increasingly interconnected digital ecosystem.
References
Adebayo, A. (2022). Contextualizing influence of organizational governance on information security risk management efficacy (Publication Number 30243867) [D.I.T., Capella University]. ProQuest One Academic.
Alecse, C. (2022). The impact of choice overload on decision deferral in cybersecurity. SAIS 2022 Proceedings. https://aisel.aisnet.org/sais2022/42
Alghamdi, N. A., & Al-Baity, H. H. (2022). Augmented analytics driven by AI: A digital transformation beyond business intelligence. Sensors (Basel), 22(20). https://doi.org/10.3390/s22208071
Alnifie, K. M., & Kim, C. (2023). Appraising the manifestation of optimism bias and its impact on human perception of cyber security: A meta analysis. Journal of Information Security, 14(02), 93-110. https://doi.org/10.4236/jis.2023.142007
Andrade, R. O., Fuertes, W., Cazares, M., Ortiz-Garcés, I., & Navas, G. (2022). An exploratory study of cognitive sciences applied to cybersecurity. Electronics, 11(11). https://doi.org/10.3390/electronics11111692
Arellano, J., Coello, P., Fucci, E., & Uliana, E. (2023). A better way: How to reduce bias and improve decision-making in multidisciplinary teams. In A. Samson (Ed.), Behavioral economics guide 2023 (pp. 26-35).
Baer, T. (2019). Understand, manage, and prevent algorithmic bias: A guide for business users and data scientists. Springer.
Balayn, A., Lofi, C., & Houben, G.-J. (2021). Managing bias and unfairness in data for decision support: A survey of machine learning and data engineering approaches to identify and mitigate bias and unfairness within data management and analytics systems. The VLDB Journal, 30(5), 739-768. https://doi.org/10.1007/s00778-021-00671-8
Bamberger, M., & Mabry, L. (2020). Realworld evaluation: Working under budget, time, data, and political constraints (3rd ed.) https://doi.org/10.4135/9781071909607
Belhadi, A., Kamble, S., Fosso Wamba, S., & Queiroz, M. M. (2021). Building supply-chain resilience: An artificial intelligence-based technique and decision-making framework. International Journal of Production Research, 60(14), 4487-4507. https://doi.org/10.1080/00207543.2021.1950935
Berry, H. S. (2023). The importance of cybersecurity in supply chain. 2023 11th International Symposium on Digital Forensics and Security (ISDFS).
Berthet, V. (2021). The impact of cognitive biases on professionals' decision-making: A review of four occupational areas. Frontiers in Psychology, 12, 802439. https://doi.org/10.3389/fpsyg.2021.802439
Binns, R. (2022). Human judgment in algorithmic loops: Individual justice and automated decision-making. Regulation & Governance, 16(1), 197-211. https://doi.org/https://doi.org/10.1111/rego.12358
Booch, G., Fabiano, F., Horesh, L., Kate, K., Lenchner, J., Linck, N., Loreggia, A., Murgesan, K., Mattei, N., Rossi, F., & Srivastava, B. (2021). Thinking fast and slow in AI. Proceedings of the AAAI Conference on Artificial Intelligence, 35(17), 15042-15046. https://doi.org/10.1609/aaai.v35i17.17765
Boyson, S., Corsi, T. M., & Paraskevas, J.-P. (2021). Defending digital supply chains: Evidence from a decade-long research program. Technovation, 102380. https://doi.org/10.1016/j.technovation.2021.102380
Burton, J. W., Stein, M. K., & Jensen, T. B. (2019). A systematic review of algorithm aversion in augmented decision making. Journal of Behavioral Decision Making, 33(2), 220-239. https://doi.org/10.1002/bdm.2155
Cains, M. G., Flora, L., Taber, D., King, Z., & Henshel, D. S. (2022). Defining cyber security and cyber security risk within a multidisciplinary context using expert elicitation. Risk Analysis, 42(8), 1643-1669. https://doi.org/10.1111/risa.13687
Carmichael, D. G. (2020). Bias and decision making – an overview systems explanation. Civil Engineering and Environmental Systems, 37(1-2), 48-61. https://doi.org/10.1080/10286608.2020.1744133
Ceric, A., & Holland, P. (2019). The role of cognitive biases in anticipating and responding to cyberattacks. Information Technology & People, 32(1), 171-188. https://doi.org/10.1108/itp-11-2017-0390
Cheung, K.-F., Bell, M. G. H., & Bhattacharjya, J. (2021). Cybersecurity in logistics and supply chain management: An overview and future research directions. Transportation Research Part E: Logistics and Transportation Review, 146, 102217. https://doi.org/10.1016/j.tre.2020.102217
Colicchia, C., Creazza, A., & Menachof, D. A. (2019). Managing cyber and information risks in supply chains: Insights from an exploratory analysis. Supply chain management, 24(2), 215-240. https://doi.org/10.1108/SCM-09-2017-0289
Cox, L. A. (2023). Natural, artificial, and social intelligence for decision-making. In AI-ML for decision and risk analysis (pp. 65-101). https://doi.org/10.1007/978-3-031-32013-2_3
Creel, K., & Hellman, D. (2022). The algorithmic leviathan: Arbitrariness, fairness, and opportunity in algorithmic decision-making systems. Canadian Journal of Philosophy, 52(1), 26-43. https://doi.org/10.1017/can.2022.3
Cucu, C., Gavrioloaia, G. P., Bologa, R. P., & Cazacu, M. (2019). Current technologies and trends in cybersecurity and the impact of artificial intelligence. https://doi.org/ 10.12753/2066-026X-21-099
Culot, G., Nassimbeni, G., Podrecca, M., & Sartor, M. (2021). The ISO/IEC 27001 information security management standard: Literature review and theory-based research agenda. The TQM Journal, 33(7), 76-105. https://doi.org/10.1108/TQM-09-2020-0202
Cybersecurity and Infrastructure Security Agency, & National Institute of Standards and Technology. (2021). Defending against software supply chain attacks. https://www.cisa.gov/sites/default/files/publications/defending_against_software_supply_chain_attacks_508_1.pdf
Deiva Ganesh, A., & Kalpana, P. (2022). Future of artificial intelligence and its influence on supply chain risk management – a systematic review. Computers & Industrial Engineering, 169. https://doi.org/10.1016/j.cie.2022.108206
Deniz, N. (2020). Cognitive biases in mcdm methods: An embedded filter proposal through sustainable supplier selection problem. Journal of Enterprise Information Management, 33(5), 947-963. https://doi.org/10.1108/jeim-09-2019-0285
Dror, I. E. (2020). Cognitive and human factors in expert decision making: Six fallacies and the eight sources of bias. Analytical Chemistry, 92(12), 7998-8004. https://doi.org/10.1021/acs.analchem.0c00704
Eggers, S. L. (2021). Towards a new supply chain cybersecurity risk analysis technique. https://www.osti.gov/biblio/1877401
Einhorn, C. S. (2023). Problem solver: Maximizing your strengths to make better decisions. Cornell University Press. https://books.google.com/books?id=5B6rEAAAQBAJ
Elangovan, K. R. (2019). Security framework for supply-chain management. In A. Awasthi & K. Grzybowska (Eds.), Handbook of research on interdisciplinary approaches to decision making for sustainable supply chains (pp. 533-555). IGI Global. https://doi.org/10.4018/978-1-5225-9570-0.ch025
Enders, T., Martin, D., Sehgal, G. G., & Schüritz, R. (2020). Igniting the spark: Overcoming organizational change resistance to advance innovation adoption – the case of data-driven services. Exploring Service Science.
Exec. Order No. 14017, 3 CFR 521. (2021). https://www.govinfo.gov/content/pkg/FR-2021-03-01/pdf/2021-04280.pdf
Fielder, A., König, S., Panaousis, E., Schauer, S., & Rass, S. (2018). Risk assessment uncertainties in cybersecurity investments. Games, 9(2). https://doi.org/10.3390/g9020034
Fischhoff, B. (2003). Hindsight ≠ foresight: The effect of outcome knowledge on judgment under uncertainty. Quality & Safety in Health Care, 12(4), 304-311. https://doi.org/10.1136/qhc.12.4.304
Fischhoff, B., & Broomell, S. B. (2020). Judgment and decision making. Annual Review of Psychology, 71, 331-355. https://doi.org/10.1146/annurev-psych-010419-050747
Fleischman, G. M., Valentine, S. R., Curtis, M. B., & Mohapatra, P. S. (2023). The influence of ethical beliefs and attitudes, norms, and prior outcomes on cybersecurity investment decisions. Business & Society, 62(3), 488-529. https://doi.org/10.1177/00076503221110156
Frietzsche, R. (2019). Doing risk management correctly. Cyber Security: A Peer-Reviewed Journal, 3(1), 14-24. https://www.ingentaconnect.com/content/hsp/jcs/2019/00000003/00000001/art00003
Ganin, A. A., Quach, P., Panwar, M., Collier, Z. A., Keisler, J. M., Marchese, D., & Linkov, I. (2020). Multicriteria decision framework for cybersecurity risk assessment and management. Risk Analysis, 40(1), 183-199. https://doi.org/10.1111/risa.12891
Gigerenzer, G. (2023). The intelligence of intuition. Cambridge University Press. https://books.google.com/books?id=7IHZEAAAQBAJ
Goel, R., Kumar, A., & Haddow, J. (2020). PRISM: A strategic decision framework for cybersecurity risk assessment. Information & Computer Security, 28(4), 591-625. https://doi.org/10.1108/ics-11-2018-0131
Hennink, M., & Kaiser, B. N. (2022). Sample sizes for saturation in qualitative research: A systematic review of empirical tests. Social Science & Medicine, 292, 114523. https://doi.org/https://doi.org/10.1016/j.socscimed.2021.114523
Hitchcox, Z. (2020). Limitations of cybersecurity frameworks that cybersecurity specialists must understand to reduce cybersecurity breaches (Publication Number 28086762) [D.C.S., Colorado Technical University]. ProQuest Dissertations & Theses Global.
Huo, L. a., Guo, H., Cheng, Y., & Xie, X. (2020). A new model for supply chain risk propagation considering herd mentality and risk preference under warning information on multiplex networks. Physica A: Statistical Mechanics and its Applications, 545, 123506. https://doi.org/https://doi.org/10.1016/j.physa.2019.123506
ISC2. (2023). ISC2 cybersecurity workforce study. https://media.isc2.org/-/media/Project/ISC2/Main/Media/documents/research/ISC2_Cybersecurity_Workforce_Study_2023.pdf
Jarjoui, S., & Murimi, R. (2021). A framework for enterprise cybersecurity risk management. In Advances in cybersecurity management (pp. 139-161). Springer. https://doi.org/10.1007/978-3-030-71381-2_8
Johnson, C., Gutzwiller, R., Ferguson-Walter, K., & Fugate, S. (2020). A cyber-relevant table of decision making biases and their definitions. https://doi.org/10.13140/RG.2.2.14891.87846/1
Johnson, C. K., Gutzwiller, R. S., Gervais, J., & Ferguson-Walter, K. J. (2021). Decision-making biases and cyber attackers. 2021 36th IEEE/ACM International Conference on Automated Software Engineering Workshops (ASEW).
Joint Task Force Transformation Initiative. (2012). Guide for conducting risk assessments (NIST SP800-30). National Institute of Standards and Technology. https://doi.org/10.6028/NIST.SP.800-30r1
Kadyschuk, L. (2023). Interpretive analysis. In J. M. Okoko, S. Tunison, & K. D. Walker (Eds.), Varieties of qualitative research methods: Selected contextual perspectives (pp. 249-255). Springer International Publishing. https://doi.org/10.1007/978-3-031-04394-9_40
Kahneman, D. (2011). Thinking, fast and slow. Farrar, Straus and Giroux.
Kahneman, D., & Frederick, S. (2005). A model of heuristic judgment. In K. Holyoak & B. Morrison (Eds.), The Cambridge handbook of thinking and reasoning (pp. 267--293). Cambridge University Press.
Kahneman, D., Lovallo, D., & Sibony, O. (2019). A structured approach to strategic decisions. MIT Sloan Management Review, 60(3), 67-73.
Kahneman, D., Sibony, O., & Sunstein, C. R. (2021). Noise: A flaw in human judgment. Little, Brown. https://books.google.com/books?id=g2MBEAAAQBAJ
Ketchen, D. J., Jr., & Craighead, C. W. (2022). Cognitive biases as impediments to enhancing supply chain entrepreneurial embeddedness. Journal of Business Logistics. https://doi.org/https://doi.org/10.1111/jbl.12307
Korolov, M. (2020). What is a supply chain attack? Why you should be wary of third-party providers. CSO (Online). https://www.csoonline.com/article/561323/supply-chain-attacks-show-why-you-should-be-wary-of-third-party-providers.html
Korteling, J. E., & Toet, A. (2022). Cognitive biases. In S. Della Sala (Ed.), Encyclopedia of behavioral neuroscience, 2nd edition (pp. 610-619). Elsevier. https://doi.org/https://doi.org/10.1016/B978-0-12-809324-5.24105-9
Korteling, J. E. H., Paradies, G. L., & Sassen-van Meer, J. P. (2023). Cognitive bias and how to improve sustainable decision making. Frontier Psychology, 14, 1129835. https://doi.org/10.3389/fpsyg.2023.1129835
Kshetri, N. (2022). Economics of supply chain cyberattacks. IT professional, 24(3), 96-100. https://doi.org/10.1109/MITP.2022.3172877
Latif, M. N. A., Aziz, N. A. A., Hussin, N. S. N., & Aziz, Z. A. (2021). Cyber security in supply chain management: A systematic review. LogForum, 17(1), 49-57.
Lee, I., & Mangalaraj, G. (2022). Big data analytics in supply chain management: A systematic literature review and research directions. Big Data and Cognitive Computing, 6(1). https://doi.org/10.3390/bdcc6010017
Li, X. (2023). New advances in social cognitive neuroscience. Scientific and Social Research, 5(8), 19-24. https://doi.org/10.26689/ssr.v5i8.5279
Lincke, S. (2024). Complying with the PCI DSS standard. In S. Lincke (Ed.), Information security planning: A practical approach (pp. 45-63). Springer International Publishing. https://doi.org/10.1007/978-3-031-43118-0_3
M'Manga, A. (2020). Designing for cyber security risk-based decision making [Doctoral dissertation, Bournemouth University]. ProQuest Dissertations Publishing.
Maheshwari, S., Gautam, P., & Jaggi, C. K. (2020). Role of big data analytics in supply chain management: Current trends and future perspectives. International Journal of Production Research, 59(6), 1875-1900. https://doi.org/10.1080/00207543.2020.1793011
McAlaney, J., & Benson, V. (2020). Cybersecurity as a social phenomenon. In V. Benson & J. McAlaney (Eds.), Cyber influence and cognitive threats (pp. 1-8). Academic Press. https://doi.org/10.1016/b978-0-12-819204-7.00001-4
Melnyk, S. A., Schoenherr, T., Speier-Pero, C., Peters, C., Chang, J. F., & Friday, D. (2022). New challenges in supply chain management: Cybersecurity across the supply chain. International Journal of Production Research, 60(1), 162-183. https://doi.org/10.1080/00207543.2021.1984606
Miles, L. A. (2021). An analysis of the combined effects of advanced information technologies and enterprise risk management on organizational performance (Publication Number 28549351) [D.B.A., Drexel University]. ProQuest One Academic.
Milkman, K. L., Chugh, D., & Bazerman, M. H. (2009). How can decision making be improved? Perspectives on Psychological Science, 4(4), 379-383. https://doi.org/10.1111/j.1745-6924.2009.01142.x
Mitchell, J. D. (2022). Behavioral supply chain management: Exploring interventions for supply chain managers (Publication Number 29998036) [D.M., Colorado Technical University]. ProQuest One Academic.
Monteiro, S., Sherbino, J., Sibbald, M., & Norman, G. (2020). Critical thinking, biases and dual processing: The enduring myth of generalisable skills. Medical Education, 54(1), 66-73. https://doi.org/10.1111/medu.13872
Murphy, M. P. A. (2021). Introduction: Uncertainty, paradoxes, and critical intuition. In Quantum social theory for critical international relations theorists: Quantizing critique (pp. 1-14). Springer International Publishing. https://doi.org/10.1007/978-3-030-60111-9_1
Naik, B., Mehta, A., Yagnik, H., & Shah, M. (2021). The impacts of artificial intelligence techniques in augmentation of cybersecurity: A comprehensive review. Complex & Intelligent Systems, 8(2), 1763-1780. https://doi.org/10.1007/s40747-021-00494-8
National Counterintelligence and Security Center. (2020). Supply chain risk management: Reducing threats to key U.S. supply chains.
https://www.dni.gov/files/NCSC/documents/supplychain/20200925-NCSC-Supply-Chain-Risk-Management-tri-fold.pdf
Panda, S. (2022). Optimal strategies for cyber security decision-making [Doctoral Dissertation, University of Surrey]. https://openresearch.surrey.ac.uk/esploro/outputs/doctoral/Optimal-Strategies-for-Cyber-Security-Decision-Making/99641066402346
Perumannil, S., & Haneef, F. (2021). Latest trends in cybersecurity after solarwind hacking attack. Journal of Cyber Security and Mobility, 1, 4.
Pignatiello, G. A., Martin, R. J., & Hickman Jr, R. L. (2020). Decision fatigue: A conceptual analysis. Journal of Health Psychology, 25(1), 123-135.
Ponto, J. (2015). Understanding and evaluating survey research. Journal of the Advanced Practitioner in Oncology, 6(2), 168-171.
Pournader, M., Kach, A., & Talluri, S. S. (2020). A review of the existing and emerging topics in the supply chain risk management literature. Decision Sciences, 51(4), 867-919. https://doi.org/10.1111/deci.12470
Rastogi, C., Zhang, Y., Wei, D., Varshney, K. R., Dhurandhar, A., & Tomsett, R. (2022). Deciding fast and slow: The role of cognitive biases in AI-assisted decision-making. Proceedings of the ACM on Human-Computer Interaction, 6(CSCW1), 1-22. https://doi.org/10.1145/3512930
Reed, R. (2020). Toward a meta-methodology for real-world problem solving [Doctoral dissertation, Stellenbosch University].
Sabillon, R. (2022). Audits in cybersecurity. In I. R. Management Association (Ed.), Research anthology on business aspects of cybersecurity (pp. 1-18). IGI Global. https://doi.org/10.4018/978-1-6684-3698-1.ch001
Santini, P., Gottardi, G., Baldi, M., & Chiaraluce, F. (2019). A data-driven approach to cyber risk assessment. Security and Communication Networks, 2019, 1-8. https://doi.org/10.1155/2019/6716918
Sawik, T. (2022). Balancing cybersecurity in a supply chain under direct and indirect cyber risks. International Journal of Production Research, 60(2), 766-782. https://doi.org/10.1080/00207543.2021.1914356
Serra, D. (2021). Decision-making: From neuroscience to neuroeconomics—an overview. Theory and Decision, 91(1), 1-80. https://doi.org/10.1007/s11238-021-09830-3
Sharp, P. B., Fradkin, I., & Eldar, E. (2022). Hierarchical inference as a source of human biases. Cognitive, Affective, & Behavioral Neuroscience. https://doi.org/10.3758/s13415-022-01020-0
Simon, H. A. (1955). A behavioral model of rational choice. The Quarterly Journal of Economics, 69(1), 99-118. https://doi.org/10.2307/1884852
Singh, T., Johnston, A. C., D'Arcy, J., & Harms, P. D. (2023). Stress in the cybersecurity profession: A systematic review of related literature and opportunities for future research. Organizational Cybersecurity Journal: Practice, Process and People. https://doi.org/10.1108/ocj-06-2022-0012
Smith, L. K. (2021). Identifying machine learning specific security controls – a qualitative exploratory study (Publication Number 28546069) [D.C.S., Colorado Technical University]. ProQuest Dissertations & Theses Global.
Snow, S. (2020). A qualitative study of strategy-driven, information security governance (ISG) (Publication Number 27960321) [D.C.S., Colorado Technical University]. ProQuest Dissertations & Theses Global.
Sumera, A., & Reddy, M. S. (2020). Behavioural biases in investing–concepts, categorization, indicators and remedial measures. RVIM Journal of Management Research, 12(1).
Terrell, S. R. (2022). Writing a proposal for your dissertation: Guidelines and examples. Guilford Publications.
Toorajipour, R., Sohrabpour, V., Nazarpour, A., Oghazi, P., & Fischl, M. (2021). Artificial intelligence in supply chain management: A systematic literature review. Journal of Business Research, 122, 502-517. https://doi.org/10.1016/j.jbusres.2020.09.009
Tversky, A., & Kahneman, D. (1974). Judgment under uncertainty: Heuristics and biases. Science, 185(4157), 1124-1131. https://doi.org/10.1126/science.185.4157.1124
van Schaik, P., Renaud, K., Wilson, C., Jansen, J., & Onibokun, J. (2020). Risk as affect: The affect heuristic in cybersecurity. Computers & Security, 90, 101651. https://doi.org/10.1016/j.cose.2019.101651
Vereschak, O., Bailly, G., & Caramiaux, B. (2021). How to evaluate trust in AI-assisted decision making? A survey of empirical methodologies. Proceedings of the ACM on Human-Computer Interaction, 5(CSCW2), 1-39. https://doi.org/10.1145/3476068
Wangen, G., Hallstensen, C., & Snekkenes, E. (2017). A framework for estimating information security risk assessment method completeness. International Journal of Information Security, 17(6), 681-699. https://doi.org/10.1007/s10207-017-0382-0
White, C. A. (2023). Mixed method exploration of cybersecurity executive decisions and cognitive bias (Doctoral dissertation]. ProQuest Dissertations Publishing.
Wolff, E. D., Growley, K., & Gruden, M. (2021). Navigating the Solarwinds supply chain attack. The Procurement Lawyer, 56(2).
Yoon, H., Scopelliti, I., & Morewedge, C. K. (2021). Decision making can be improved through observational learning. Organizational Behavior and Human Decision Processes, 162, 155-188. https://doi.org/10.1016/j.obhdp.2020.10.011
Zhang, M., Nazir, M. S., Farooqi, R., & Ishfaq, M. (2022). Moderating role of information asymmetry between cognitive biases and investment decisions: A mediating effect of risk perception. Frontier Psychology, 13, 828956. https://doi.org/10.3389/fpsyg.2022.828956
Appendix
Scenario 1: CollaboraSync is a Software as a Service (SaaS) based collaboration tool vendor renowned for its state-of-the-art real-time document editing and project management capabilities. The CIO has been friends with the founder for many years and has previously served in an advisory role with CollaboraSync. The tool allows employees to work together seamlessly, regardless of location. The organization wants to use CollaboraSync to streamline communications and project workflows among its diverse and dispersed workforce. The data shared with CollaboraSync would include employee communications, project documents, schedules, and technical information about products and services. CollaboraSync has become an integral part of a similar organization’s operations, making real-time collaboration possible and ensuring projects stay on track. CollaboraSync's tool was pivotal in managing a crucial project that significantly outpaced the expected timelines, setting a new standard in operational efficiency within a similar organization.
Scenario 2: PaySphere is a third-party payment processor facilitating seamless transactions between organizations and customers. With a robust anti-fraud system, PaySphere processes payments securely while ensuring compliance with financial regulations. The organization is looking to use PaySphere to handle all customer transactions, which include processing credit card payments, managing subscriptions, and handling refunds. The data shared with PaySphere encompasses customer financial information, transaction histories, and billing addresses. The vendor maintains PCI-DSS compliance and has provided a certificate to validate the certification. PaySphere has been a reliable partner for the organization for over a decade, handling millions of transactions amounting to over $200 million in processed payments. This long-term relationship has built a strong trust and familiarity, with PaySphere often going the extra mile to customize its solutions to meet the organization’s evolving needs.
Scenario 3: InsightAI is a cutting-edge AI tool vendor specializing in predictive analytics and machine learning solutions. The tool analyses vast amounts of data to generate insights that aid decision-making and planning. The CEO and several Executives believe InsightAI can help scrutinize market trends and consumer behavior, enabling more precise targeting in its marketing efforts. The data shared with InsightAI includes sales figures, customer feedback, and market research data. One notable use case was when a competitor organization leveraged InsightAI’s predictive analytics to forecast a sudden market shift, allowing them to adapt their strategy promptly. The action led to retaining and growing their market share when competitors faced significant losses. InsightAI’s tool was praised for its accuracy and the competitive advantage it provided, creating a strong endorsement for its capabilities within the organization.
© 2024 Dustin S. Sachs. This work is licensed under Attribution-NoDerivatives 4.0 International. To view a copy of this license, visit http://creativecommons.org/licenses/by-nd/4.0/